The ABC Company optimizes the click rate.
Introduction and Business Understanding
The ABC company wants to optimize the response (click rate) to their online ads. 13 factors were identified as potentially affecting the clock rate. We start by using the file "DOE_Click," which contains the 13 factors and two levels we should consider.
The goal is to determine which response factors will increase the click rates on ABC Company'sCompany's online ads.
Data Understanding
ABC company identified 13 factors that will be included in a 20 run Blackett-Burman design. Each element is on two levels, and the response is of continuous modeling type to count the number of clicks. Appendix A.
Analysis
Several DOE designs were considered using all 13 factors, with the most common the Screening Designs platform under the Classical Design option to create a design matrix. We should select a design with the number of runs. A complete factorial design is not realistic as it will contain 213 runs, too many, better not to run a lot, as it costs much money to complete that many runs. JMP provides multiple options to reduce the design to a fractional factorial, including minimum runs, default runs, and user selection. The minimum requirement is simply several factors plus the mean. The minimum represented the number of factors plus one, which is 14 experiments. The default design in JMP provided us with 16 runs fractional factorial plan, which meets the minimum run requirement, which is more than 14 experiments and is of resolutions ""3 main effects"", which provide the estimation of all 13 main effects, and the overall mean free of confounding with each other.
Both 14 and 16 run options were evaluated in the distributions platform, and It was determined that 20 runs would be a less costly, balanced design. Two 'factors' interactions are confounded with the main effects.
Identifying Statistically Significant Factors
After selecting a design, the data file was used to determine the statistically significant factors at a significance level of p=0.05. We performed two-level screening under the DOE platform to analyze the design. Four elements (Discount, Advertising Chanel, Color Schema, and Message Type) were statistically significant below 0.05, Appendix B. Only Discount and Ads. Channel has a Simultaneous value of less than 0.05. The remaining 16 factors had a p-value greater than 0.14, which makes them all not statistically significant. The model was run with the four selected factors to evaluate their relationship to the response of several clicks. Appendix C. All four main effects were statistically significant at p less than 0.05.
Confounding
Four top factors are unconfounding with each other, but Discount*Ads. Chanel is confounding with some of the main effects, but we can't see which one, so we have to look at the design, which action it is confounding with, and the confounding structure. We want to determine if their 2-factor interaction of the two most significant factors is confounded with the other weaker main effects. The Alias Matrix Appendix D. shows that the 2-factor exchange of Discount and Advertising Chanel are strongly cofounded with the Color Scheme indicated by the value of 0.6, and the second one is Message type with 0.2 indications.
We look at the significant values in the positive or negative directions. The sigh is not essential, but the absolute value is, and if it is a relatively large number, if it's zero, there is no confounding between the 2-factors, which are the 2 top rows, and the main effects of the first column. There are other large numbers in Discount with Color Scheme being strongly confounded with Advertising Chanel and 2-factor interaction between Ad. Chanel with Color Scheme that confounded with Discount, but they are likely to be small interactions.
Reduced Design
Based on the findings from the Alias Matrix, a new model was created, including the 2-factor interaction Discount with Ads... Chanel with four main effects, which were determined in the first run. Appendix E. Color Scheme and Discount Ads. Chanel both have p larger than 0.05 making them not statistically significant. Appendix F. Discount*Ads. Is the factor that is responsible for the Color Scheme that contributes to the effect.
We take out Color Scheme as it has the lowest effect. Appendix G. It is out the final result as all main products are statistically significant at p smaller than 0.05. Dominant factors are Discount and Ads. Chanel, they in action with Discount Ads. Chanel. In Appendix H. it shows that the effect of when we have Ads Chanel A, and we added Discount to add A so that it will increase in the click rate, but if we choose Ad B with Discount, it will increase even more compared to Ad A, it is interaction, the effect of one variable is different depending on what the level of the other variable is on the graph.
Conclusion
Screening designs were used to determine which response factors that will increase the click rates.
A fractional factorial design was used to limit the number of runs which would lower cost while still providing enough runs to estimate.
The first 20-run design identified the four most statistically significant factors, and an inspection of the alias matrix indicated that one of the main effects was confounded with the 2-factor interaction, the two most considerable main effects. Interaction plot shows us that switching Ad. Chanel from A to B has a significant impact when there is a discount – YES. Furthermore, the experiment shows that the most click responses occurred when a deal was offered and Ad Canel B (No).
Research Provided by Andrey Fateev
Appendix A. Factors and Levels
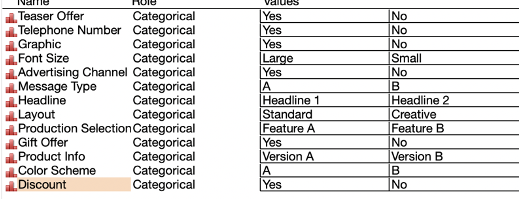
Appendix B. Analysis Result
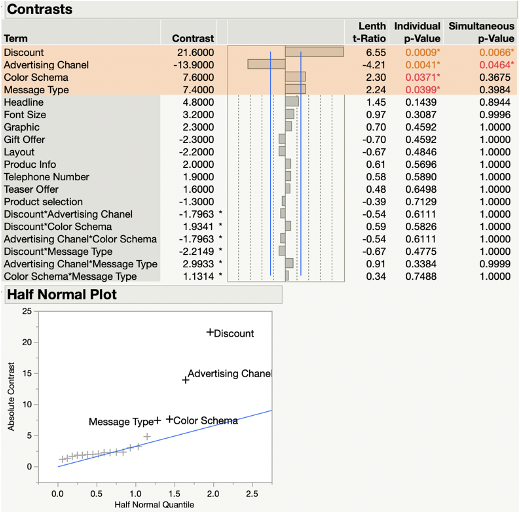
Appendix C.
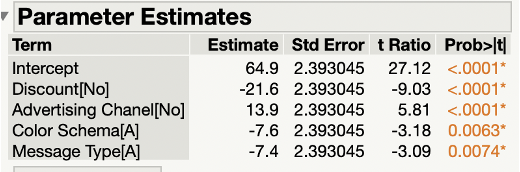
Appendix D. Alias Matrix.

Appendix E.
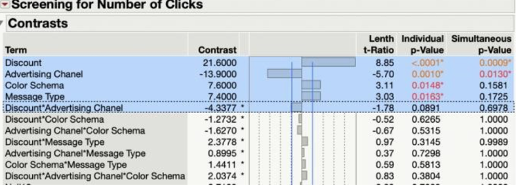
Appendix F. Results of the 2nd run.
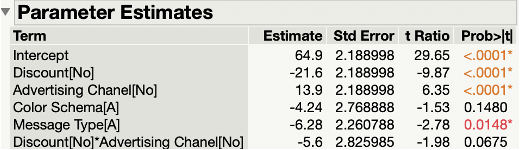
Appendix G. Final Model without Color Scheme.

Appendix H. Interaction Plot for the Final Model.

Comments